Table of Content
TABLE OF CONTENTS
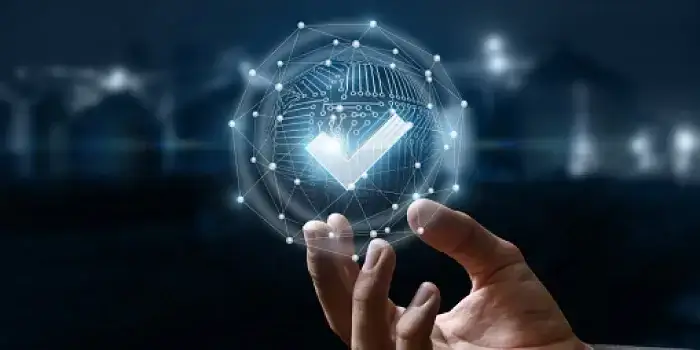
Data Quality - Overview
Corporations have started to realize that Data accumulated over the years is proving to be an invaluable asset for the business. The data is analyzed, and strategies are devised for the business based on the outcome of Analytics. The accuracy of the prediction and hence the success of the business depends on the quality of the data upon which analytics is performed. So, it becomes all the more important for the business to manage data as a strategic asset and its benefits can be fully exploited.
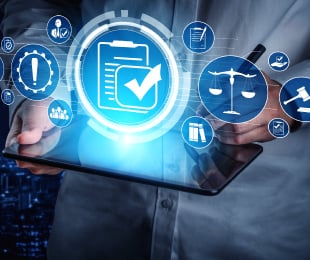
This blog aims to provide a blueprint for a highly successful Data Quality project, practices to be followed for improving Data Quality, and how companies can make the right data-driven decisions by following these best practices.
Source Systems and Data Quality Measurement
To measure the quality of the data, third-party “Data Quality tools" should hook on to the source system and measure the Data Quality. Having a detailed discussion with the owners of the systems identified for Data Quality measurement needs to be undertaken at a very early stage of the project. Many system owners may not have an issue with allowing a third-party Data Quality tool to access their data directly.
However, some systems will have regulatory compliance, because of which the systems’ owners will not permit other users or applications to access their systems directly. In such a scenario, the systems owner and the Data Quality architect will have to agree upon the format in which the data will be extracted from the source system and shared with the Data Quality measurement team for assessing the Data Quality.
Some of the Data Quality tools that are leaders in the market are Informatica, IBM, SAP, SAS, Oracle, Syncsort, Talend.
The Data Quality Architecture should be flexible enough to absorb the data from such systems in any standard format such as CSV, API, and Messages. Care should be taken such that the data that is being made available for Data Quality measurement is extracted and shared with them in an automated way.
Environment Setup
If the Data Quality tool is directly going to connect to the source system, evaluation of the source systems’ metadata across various environments is another important activity that should be carried out in the initial days of the Data Quality Measurement program. The tables or objects that hold the source data should be identical across different environments. If they are not identical, then decisions should be taken to sync them up across environments and should be completed before the developers are onboarded in the project.
If the Data Quality Team is going to receive data in the form of files, then the location in which the files or data will be shared should be identified, and the shared location is created with the help of the infrastructure team. Also, the Data Quality tool should be configured so that it can READ the files available in the SHARED Folder.
Data Quality Measurement Architecture
Figure 1: Data Quality Measurement Architecture
Data Acquisition
The architecture for the Data acquisition layer (How do we get the data for DQ Measurement) should be documented and socialized with the Business users, Data Owners, and Data Stewards. The Data Quality tool's connectivity to different source systems developed on disparate technologies should be established. The systems that don’t allow ETL tools to access the data directly should be able to send / FTP data to a shared folder.
The data which is not directly available for consumption of Data Quality Measurement should be delivered in an agreed-upon format. The Data Quality Team will validate their conformity before they are consumed. If the files don't comply with the agreed-upon format, the Data Acquisition and the subsequent Data Quality measurement should not be kicked off. An intimation or communication in the form of mail should be triggered to the team that is responsible for the source data. A similar communication protocol should be implemented for failure at any stage of the Data Quality measurement.
End-to-End Automation
The entire process of Data acquisition, Data Quality Measurement, Report generation, and Report Distribution through Data Quality Dashboard refresh should happen with zero manual intervention. Similarly, the decision to hold or purge data, namely the Source Data received in the form of a file and the data used for Data Quality measurement, also needs to be documented and agreed upon with the stakeholders. The purging process should also be automated and scheduled to happen at agreed-upon intervals.
Difference Reports and Data Quality Dashboards
In the ecosystem of applications, there will be a few attributes that traverse across systems. For example; Opportunity ID created in POS traverses to other systems. The Data Quality rule for those attributes will be the same in all the systems they are found in. Landscaping of all the source systems should be conducted, and an inventory of such attributes traveling across systems should be created. Reusable components should be built to measure the Data Quality of those attributes.
The Data Stewards will be interested in knowing the degree of consistency and integrity of a domain's data across the landscape. This will be used as an instrument by the business users to support any decisions made as part of the Data Quality Measurement program on enhancing the data or making other decisions on the content and validity of the data.
This landscaping activity will help the architecture team to create two important assets which will be used by the Data Stewards and business users – Data lineage and Data Dictionary.
Conclusion
Data Quality is more of a business problem to solve than a technical problem. The success of a Data quality program depends on the synergy between the Data Stewards, Application Owners, and IT Teams. The winning team will have the right combination of representatives from each of the teams, along with representation from the Project Sponsor and business owners.
Tags
Data in Motion
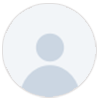
M.S Udankar
He has 14 years of IT experience in Data Warehouses, Data Integration, Data Quality and Business Intelligence Consulting Space for marquee clients.