Table of Content
TABLE OF CONTENTS
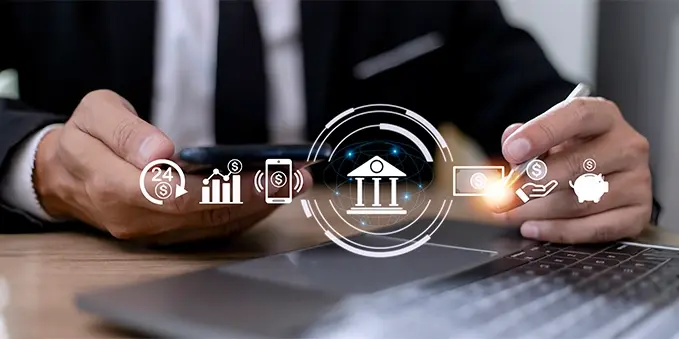
The financial landscape is undergoing a seismic shift. Customer expectations are evolving, competition is fiercer than ever, and data is the new gold. To stay ahead, banks need to transform from data repositories to data-driven powerhouses. This is where Artificial Intelligence (AI) and Machine Learning (ML) technologies are revolutionizing how banks analyze data, enhance customer experiences, mitigate risks, and streamline operations. To thrive in the AI-powered digital age, banks must adopt an AI and analytics capability stack that delivers intelligent, personalized solutions and distinctive experiences at scale in real-time.
Uncovering Customer to Customer Relationships with AI/ML
Watch now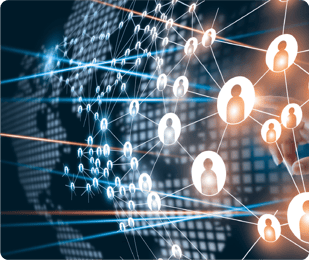
Why do banks need to embrace AI and ML?
The banking sector, traditionally a bastion of stability, now faces a maelstrom of change. Customers demand more, competition is fierce, and the data landscape is vast. Additionally, banks and financial institutions are sitting on a goldmine of data. This data, when effectively analyzed, can provide deep insights into customer behavior, market trends, and operational inefficiencies. AI and ML enable banks to transform this data into actionable intelligence, offering several strategic advantages:
- Enhanced customer experience: AI-driven insights help banks deliver personalized services and offers, thereby improving customer satisfaction and loyalty.
- Fraud prevention and risk management: AI and ML models can detect fraudulent activities and assess credit risks with greater accuracy, reducing potential losses.
- Operational efficiency: Automation of routine tasks through AI and ML can significantly increase productivity and reduce costs. A McKinsey report predicts that GenAI has the potential to supercharge banking productivity by up to 5%.
Imperative for AI-first strategy
To stay competitive and relevant, traditional banks must shift towards an AI-first strategy. This involves integrating AI across all aspects of their operations, from customer service to backend processes. The economies of scale achieved through the efficient deployment of AI technologies compel banks to enhance customer engagement with distinctive experiences and superior value propositions. This transformation begins with intelligent, highly personalized offers and extends to smart services, streamlined omnichannel journeys, and seamless integration of trusted bank functionalities within partner ecosystems.
Benefits of AI and ML in the banking industry
AI and ML are set to redefine banking operations and customer interactions. According to Autonomous, banks worldwide can reduce costs by 22% by 2030 with AI technologies, with potential savings reaching $1 trillion. Here are the key areas where AI and ML are making a significant impact:
- Fraud detection: It is a critical area where AI and ML are proving invaluable. Traditional rule-based systems often fail to detect sophisticated fraud schemes. AI models, however, can analyze vast amounts of data in real-time to identify unusual patterns and flag potential frauds. This proactive approach not only prevents fraud but also enhances customer trust.
- Personalized customer service: AI-powered chatbots and virtual assistants provide personalized customer service, answering queries and offering tailored financial advice around the clock. By analyzing customer data, AI systems can recommend products and services that match individual needs, thereby increasing customer engagement and satisfaction.
- Credit risk management: Accurately assessing credit risk is crucial for financial stability. AI and ML algorithms can analyze historical data, transaction patterns, and even social media behavior to predict a customer’s creditworthiness more accurately than traditional methods. This leads to better decision-making and reduced default rates.
- Compliance and regulatory reporting: Banks face stringent regulatory requirements and compliance challenges. AI can automate the data collection and reporting processes, ensuring accuracy and saving significant time and resources. Machine learning algorithms can also monitor transactions and activities to ensure compliance with regulatory standards, reducing the risk of fines and penalties.
- Data analysis and insights: AI and ML enable banks to extract actionable insights from vast datasets. Predictive analytics help forecast market trends, customer behavior, and potential risks. These insights allow banks to make informed decisions, optimize operations, and develop targeted marketing strategies.
- Streamlined operations: AI and ML can automate repetitive tasks like loan application processing, account management, and data entry, freeing up valuable human resources for more complex tasks.
- Increased productivity and cost savings: Automation of routine and repetitive tasks using AI and ML can drastically increase productivity. Processes such as loan approvals, customer onboarding, and document verification can be automated, freeing up human resources for more complex tasks. This not only speeds up operations but also reduces costs.
Challenges in implementing AI and ML in banking
Despite the clear benefits, implementing AI and ML in banking comes with its own set of challenges:
- Data privacy and security: Handling sensitive customer data requires robust security measures. Banks must ensure compliance with data protection regulations and safeguard against cyber threats.
- Integration with legacy systems: Many banks still operate on outdated legacy systems that are not compatible with modern AI technologies. Upgrading these systems can be costly and time-consuming.
- Talent shortage: There is a significant shortage of skilled professionals who can develop and manage AI and ML models. Banks need to invest in training and hiring to bridge this gap.
- Regulatory compliance: Navigating the complex regulatory landscape while implementing AI solutions can be challenging. Banks must work closely with regulators to ensure compliance and mitigate risks.
Overcoming challenges with AI and ML
To address these challenges, banks can take several steps:
- Invest in robust cybersecurity measures: Ensuring data privacy and security should be a top priority. Banks must invest in advanced cybersecurity technologies and establish strict data governance policies.
- Modernize IT infrastructure: Upgrading legacy systems to support AI and ML technologies is essential. Banks should consider scalable and flexible cloud-based solutions to facilitate integration and innovation.
- Develop a skilled workforce: Investing in training programs and hiring skilled professionals can help banks build a competent workforce capable of effectively leveraging AI and ML technologies.
- Collaborate with regulators: Engaging with regulators to understand compliance requirements and integrating these into AI implementation strategies can help mitigate regulatory risks.
Conclusion
AI and ML are transforming the banking industry, offering unprecedented opportunities for growth, efficiency, and customer satisfaction. By embracing these technologies, banks can not only stay competitive but also set new benchmarks in innovation and customer service. However, the journey toward becoming an AI-first bank requires overcoming significant challenges, from data privacy concerns to upgrading legacy systems.
With strategic investments and a proactive approach, banks can harness the full potential of AI and ML, driving the future of banking toward a more intelligent and customer-centric paradigm. If you want to explore the power of data-driven insights in transforming banking experiences, watch our on-demand webinar now. Register now!
Related Webinar
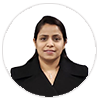
Deepti Soni
Director, Data Science and Analytics
Deepti Soni is a Data Science professional with more than ten years of experience in architecting innovative analytical solutions for strategic business problems.