Table of Content
TABLE OF CONTENTS
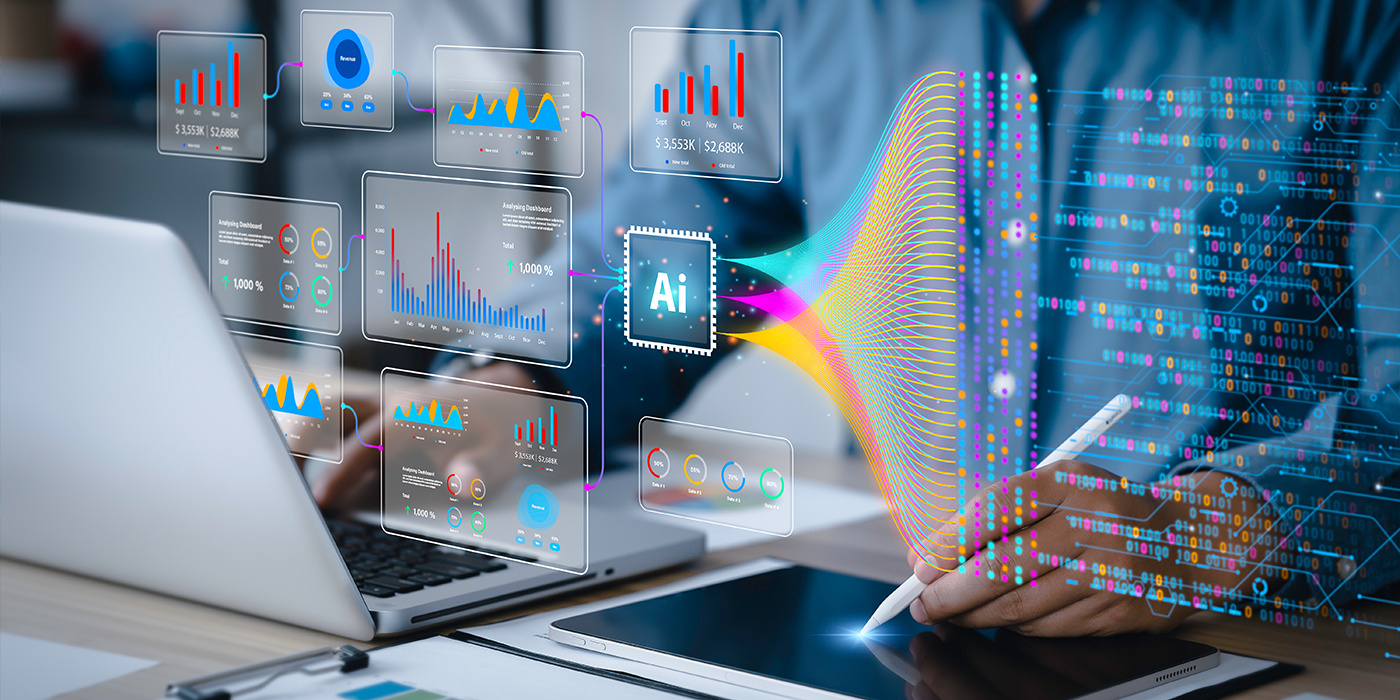
Master Data Management (MDM) systems ensure organizations maintain consistent, accurate, and reliable data. However, as the volume and complexity of data grow, maintaining data quality and stewardship becomes increasingly challenging.
Generative AI (GenAI) offers a transformative approach to address these challenges. By enhancing existing MDM solutions, GenAI enables organizations to manage their data more efficiently and effectively. MDM systems can leverage AI algorithms to automate and optimize data cleansing and enrichment processes. GenAI can identify and intelligently merge duplicate records, making MDM more efficient and scalable.
The pivotal role of GenAI in enhancing MDM
In traditional MDM systems, data cleansing and enrichment are often governed by a fixed set of rules. These rules might include validating email formats, ensuring phone numbers match a specific pattern, or checking for certain mandatory fields. While these rules are helpful, they are rigid and lack the ability to adapt to the complexity and variability of real-world data.
GenAI goes beyond these limitations by using machine learning to identify and correct data inconsistencies dynamically. It can handle complex scenarios where traditional rules fall short, such as resolving variations in customer names, addresses, or transaction histories. GenAI also assists in data stewardship by providing intelligent recommendations, significantly reducing the burden on human data stewards. This empowerment allows data stewards to focus on more strategic tasks while ensuring cleaner, more reliable master data.
AI can significantly improve data quality and stewardship by automating repetitive, error-prone tasks and providing more intelligent ways to enrich data.
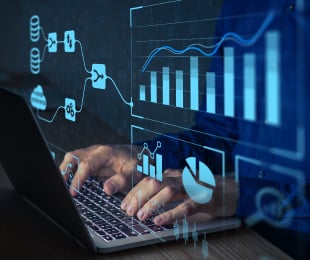
Empowering IBM MDM and Data Stewardship Center with GenAI
IBM's MDM and Data Stewardship Center are potent tools that help organizations manage their master data. By integrating GenAI into these platforms, organizations can unlock advanced capabilities, such as real-time data quality insights, automated suspect matching, and data enrichment. Gen AI can support data stewards by automating many of their manual tasks. This collaboration not only improves efficiency but also enhances the quality of master data, resulting in better decision-making and operational performance.
Key use cases of GenAI in MDM
Integrating GenAI with MDM systems opens up many possibilities for improving data quality and stewardship.
Here are some key use cases where AI can make a significant impact:
Data cleansing: From manual effort to AI automation
Data cleansing is a critical aspect of MDM. It involves identifying and correcting data inaccuracies, inconsistencies, and errors. Historically, this has been a labor-intensive task, requiring significant human oversight. However, GenAI has the potential to transform this by automating the identification of common data quality issues such as duplicate records, missing values, and inconsistencies. It can automatically detect outliers or errors and suggest corrections, reducing manual effort and improving data processing speed.
Data enrichment and standardization: Filling gaps and adding context
Data enrichment enhances the value of existing data by adding missing information, validating records, and integrating external data sources. Generative AI can fill in missing data by analyzing patterns, making intelligent predictions, or imputing these values based on historical patterns or external knowledge bases. It can also standardize data formats, ensuring consistency and improving data interoperability.
Interpretative suspect matching and recommendation
AI-powered models enhance suspect-matching processes by improving the accuracy and explainability of matching processes. GenAI can go beyond simply suggesting a match by explaining why two entities (e.g., customer records) are likely the same, considering factors like name variations, address discrepancies, or transaction history. This interpretative capability helps data stewards make more informed decisions about resolving duplicates and handling non-matching attributes, improving the overall trust in the matching process.
Improving efficiency with AI-powered data stewardship
While essential for data quality, data stewards can significantly benefit from generative AI's capabilities to perform mundane tasks and offer intelligent insights. AI is a valuable "co-pilot," enhancing the data stewardship process by suggesting corrective actions, identifying issues, and providing real-time feedback.
Reducing human error and enhancing decision-making
AI-powered tools can automate many data stewardship tasks, such as data cleansing, validation, and anomaly detection. This reduces the risk of human error and allows data stewards to focus on data quality initiatives and governance.
The role of AI as a co-pilot for data stewards
By analyzing data, identifying inconsistencies, and offering intelligent recommendations, GenAI enhances the decision-making process. It enables human data stewards to make more informed decisions, especially in complex cases involving suspect matching or data enrichment. This AI-human collaboration is key to achieving more efficient and accurate MDM processes.
Seamless integration of GenAI into MDM architecture and workflows
For organizations to fully realize the benefits of GenAI in MDM, it's essential to understand how AI can be integrated into existing MDM architectures and workflows. IBM's Master Data Management (MDM) platform provides a robust framework for managing enterprise-wide master data, while its Data Stewardship Center ensures effective governance and data stewardship.
GenAI can seamlessly integrate into MDM platforms using external APIs. These APIs enable continuous, real-time data analysis and automated insights without disrupting existing workflows. For example, GenAI-powered APIs can identify errors such as duplicates or missing fields and suggest intelligent corrections as data flows through the system. This feedback loop ensures that master data remains reliable and accurate while reducing manual intervention and improving the efficiency and reliability of data governance across the organization.
What the future holds for MDM
The future of MDM lies in the deeper integration of advanced technologies like GenAI. As AI continues to evolve, its capabilities in data management will only grow, offering new opportunities for innovation and efficiency.
Generative AI is just the beginning. Future innovations could include more advanced AI models that can autonomously manage data, predict trends, and provide strategic insights to organizations. However, it's important to note that with these advanced capabilities, there may be potential risks, such as data privacy concerns or the need for human oversight to ensure the ethical use of AI. The possibilities are vast, and the future of MDM is bright with AI-driven innovations, offering hope for a more efficient and insightful data management future.
Conclusion
AI-powered features within MDM systems, including automated data profiling, anomaly detection, and real-time data quality monitoring, can significantly improve data governance and decision-making. As AI advances, the opportunities for innovation in MDM are limitless, paving the way for a future where data is more reliable, accurate, and valuable than ever.
Want to learn more about MDM modernization and how it can transform your data management strategy? Watch our on-demand webinar.
Related Webinar
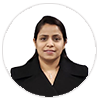
Deepti Soni
Director, Data Science and Analytics
Deepti Soni is a Data Science professional with more than ten years of experience in architecting innovative analytical solutions for strategic business problems.