Table of Content
TABLE OF CONTENTS
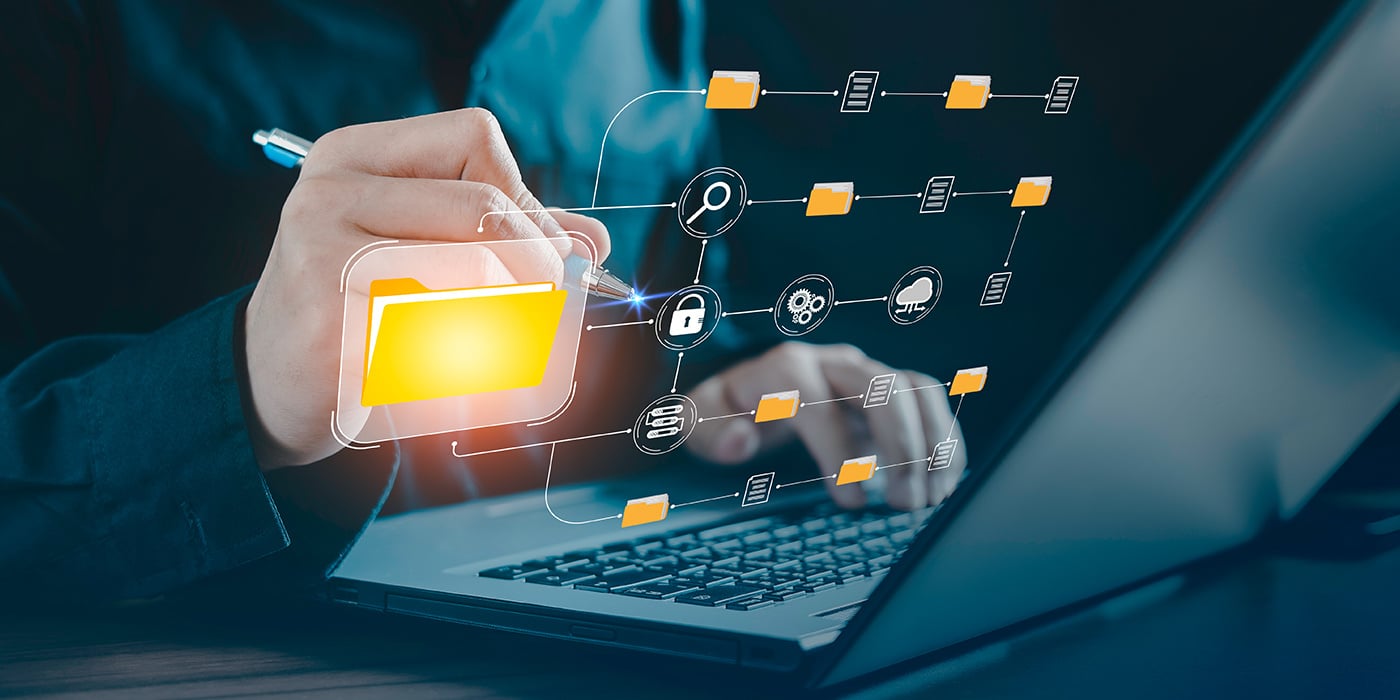
In the era of technological advancements, businesses turn to automation to streamline their operations and gain a competitive edge. However, embarking on an automation journey may not be as easy as it may seem. With the rise of automation, the role of data management has become highly crucial.
Today, data is the lifeblood of any organization, and to ensure it remains accurate, up-to-date, and valuable in this rapidly changing environment, businesses must adopt robust strategies.
As more and more organizations embrace automation, the challenges and opportunities surrounding data management evolve rapidly. As we delve into data management in an automated world, it's important to understand the challenges that come with it.
The challenges of data management in an automated world
Automation holds the promise of efficiency and real-time insights, but it also introduces new complexities to data management:
- Data volume and complexity: The volume of data being generated today is staggering. Automation allows for continuous data collection, but managing this massive inrush can become overwhelming. Data comes from a wide range of sources—structured and unstructured, internal and external—and combining them into a cohesive system is complicated. This complexity can lead to inconsistencies and inaccuracies in data without proper management.
- Integration across systems: As businesses adopt more automation tools, they often find themselves juggling different systems that don't always communicate well with each other. Integrating these systems to ensure data flows seamlessly across the organization can take time and effort. Without proper integration, data silos form, which can lead to fragmented insights and missed opportunities.
- Maintaining data quality: Even with automation, ensuring the quality of data—its accuracy, completeness, and consistency—remains a top concern. Automation can help detect and correct some errors, but if not implemented correctly, it can also perpetuate issues, leading to unreliable data. Automation at scale can magnify minor mistakes, turning them into significant problems.
Opportunities automation brings to data management
While automation does present its fair share of challenges, it also opens up a world of opportunities for data management.
- Streamlining data workflows: Automation can significantly improve the efficiency of data workflows. Tasks that once required manual intervention, such as data collection, validation, and reporting, can now be automated, freeing up valuable time for data teams to focus on strategic analysis and decision-making. Automation allows for faster data processing, meaning the business can quickly make data-driven decisions.
- Enhancing data accuracy with AI and ML: Advanced algorithms powered by artificial intelligence (AI) and machine learning (ML) can improve data accuracy. These technologies can automatically identify patterns in data, flagging anomalies or discrepancies that could be missed by human oversight. ML models can continuously refine themselves, ensuring better data quality over time.
- Real-time insights and integration: Automation enables real-time data updates and insights, allowing businesses to react quickly to market trends, customer behaviors, or operational shifts. Systems can continuously monitor incoming data, ensuring teams constantly use the most current information. This real-time capability is critical in retail, healthcare, and finance, where timing is crucial for decision-making.
How to stay ahead of the competition
As the role of automation grows, businesses must evolve their data management strategies to stay ahead. Here are some best practices:
- Implement proactive data governance: Data governance is essential to ensuring data remains accurate and compliant with regulations. Implementing governance frameworks adaptable to automation can help you stay on top of the growing volume of data. Proactive data governance includes establishing data ownership, security, and accountability policies and using automation tools to enforce these policies.
- Leverage automation for continuous monitoring: Automation isn't just for collecting and analyzing data—it's also for continuously monitoring the health of data systems. Automated monitoring tools can flag data discrepancies, incomplete records, or breaches in real-time, allowing immediate corrective action.
- Upskill your data teams: As automation plays a larger role in data management, the skills needed to oversee these systems are also evolving. Upskilling your team in areas like AI, ML, and data automation tools ensures they can effectively manage the changing landscape. Businesses that invest in employee training will be better equipped to leverage automation tools to their full potential.
- Real-time validation mechanisms: Set up systems that automatically validate incoming data in real-time. This prevents inaccurate or incomplete data from entering the systems in the first place. By catching errors as they occur, you reduce the need for time-consuming data-cleaning efforts later.
- Automate data hygiene: Routine data hygiene—tasks like deduplication, formatting, and correction—can be automated to ensure the data remains clean. Automated data hygiene workflows ensure errors are caught and corrected regularly, keeping data in shape.
- Build a scalable data infrastructure: As automation increases, so does the need for a scalable data infrastructure. Ensure that your systems can handle large volumes of data without compromising performance. A scalable infrastructure allows seamless data management as the business grows and automation efforts expand.
The future of data management in automation
The role of automation in data management will only grow in the future. AI and machine learning are expected to become even more powerful, allowing more profound insights and refined data processes. Automation will continue to reduce the burden of manual tasks, but it will require ongoing adaptation. Businesses that are prepared to embrace new tools, systems, and strategies will find themselves well-positioned to lead in this new era of data management.
Conclusion
The global enterprise data management market is poised for substantial growth, projected to increase by over 200% from 2024 to 2034. The market is expected to reach $349.52 billion by 2034, from $111.44 billion in 2024. The future of data management will require businesses to be agile and forward-thinking to effectively manage, protect, and utilize their data assets.
Related Webinar

Michael Ashwell
VP and GM Data Management, Mastech InfoTrellis
Michael is a seasoned professional with over 35 years of experience in enterprise architecture, solution development, cloud offerings, global sales, and consulting. He spent 30+ years at IBM where he held various roles, including leading the Data and Analytics Lab Services Cloud COE, and developed several key offerings.