Table of Content
TABLE OF CONTENTS
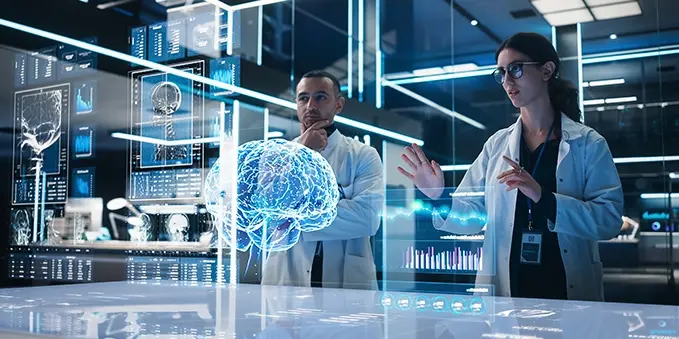
Picture a doctor who can access your complete health history, not just from their office but every healthcare facility you've ever been to. This isn't a futuristic dream. It's the reality that artificial intelligence (AI) and machine learning (ML) are bringing to healthcare. These technologies unify fragmented patient data, making it easier for healthcare professionals to provide comprehensive care.
Despite the rapid pace of medical advancements, the data that drives them often remains scattered and isolated. Each patient's journey generates a wealth of information, from electronic health records to lab results and imaging systems. The challenge lies in connecting these disparate data points into a cohesive and comprehensive picture of the patient's health, a challenge that Master Data Management (MDM) is uniquely equipped to address. This challenge is not a distant problem, but a pressing issue that healthcare organizations need to address now.
MDM ensures data integrity, consistency, and accessibility across all healthcare platforms. AI/ML-powered MDM transforms fragmented data into actionable insights, improving patient care, operational efficiency, and clinical outcomes.
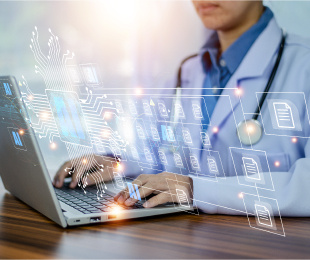
The problem - fragmented patient data
The fragmented data scenario, unfortunately, is the reality for many patients today. Their medical information gets siloed within various healthcare providers' facilities and IT systems. The ramifications of fragmented patient data are manifold and extend across the spectrum of healthcare delivery:
- Inaccurate diagnoses: Without access to comprehensive, up-to-date medical histories and test results, healthcare providers will struggle to make accurate diagnoses. Fragmented data can lead to oversight of critical information, such as allergies, previous conditions, or medication interactions, potentially compromising diagnostic accuracy.
- Ineffective treatment plans: Effective treatment decisions hinge on a holistic view of a patient's health profile. Fragmented data makes it difficult for healthcare professionals to formulate comprehensive treatment plans tailored to patients' needs. Incomplete information may result in inappropriate or suboptimal treatment choices.
- Wasted resources: When healthcare providers lack access to a patient's medical history, tests and procedures are often duplicated. This redundancy not only wastes valuable time and resources but also exposes patients to unnecessary risks and discomfort.
- Poor patient experience: From a patient's perspective, dealing with fragmented data translates into inconvenience, repetitive paperwork, and bad experiences. Continuity of care is disrupted when patients need to repeatedly provide the same information to different providers, leading to frustration and a diminished overall experience.
Master Data Management to the rescue
MDM ensures the uniformity, accuracy, stewardship, semantic consistency, and accountability of the enterprise's official shared master data assets. In simpler terms, it's about centralizing data from various sources to create a single, reliable source of truth. This involves integrating patient data from different systems into one unified, comprehensive view. The master data typically includes:
- Patient demographics
- Medical history
- Procedures and surgeries
- Lab results and imaging studies
The benefits of MDM in healthcare
- Improved data accuracy and consistency: By eliminating duplicates and inconsistencies, MDM enhances the reliability of patient information, reducing errors and ensuring that healthcare decisions are based on trustworthy data.
- Enhanced patient care coordination: Healthcare providers across different specialties can collaborate more effectively with a unified patient view. This coordination is crucial for managing chronic conditions or complex cases involving multiple specialists.
- Better clinical decision-making: Clean and consistent data allows AI and ML tools to generate more accurate diagnoses, predict potential health risks, and recommend personalized treatment plans.
- Reduced healthcare costs: MDM helps cut costs for patients and healthcare providers by preventing duplicate data and ensuring data accuracy.
How AI/ML supercharges MDM
MDM provides a solid foundation for organizing and managing critical data. However, MDM can be further enhanced by the power of AI and ML. In this context, AI/ML is not a replacement for MDM but a tool that can be integrated into MDM to enhance its capabilities. Integrating AI into MDM helps healthcare providers achieve goals faster, improves customer experience, and results in a higher return on investment (ROI). This combination creates what's known as Smart MDM, a game-changer for data-driven excellence in healthcare. Smart MDM automates and enhances various data management processes to make more intelligent and responsive systems.
How AI/ML can be used within MDM
- Identifying and resolving data inconsistencies: AI/ML can analyze vast amounts of data to identify duplicates, errors, and missing information.
- Automating data cleansing and enrichment: Cleaning and enriching patient data is time-consuming and error-prone. AI/ML can automate these tasks by identifying missing elements and suggesting appropriate data points to fill the gaps.
- Extracting valuable insights from patient data: AI and machine learning can analyze massive amounts of data, like patient records and scans, to find hidden patterns. This helps spot trends in disease outbreaks, predict patient risks, and even identify early signs of illness.
- Predicting health risks and personalizing treatment plans: Predictive analytics is one of the most transformative applications of AI and ML in healthcare. By analyzing factors like medical history, lifestyle habits, and genetic data, AI algorithms can identify patients at a higher risk of developing certain diseases.
The future of AI/ML and MDM in healthcare
The synergy between AI/ML and MDM holds immense promise for revolutionizing healthcare delivery. Here's a glimpse into the exciting possibilities that lie ahead:
- More accurate and personalized diagnostics: AI algorithms, armed with high-quality, unified patient data, can analyze medical scans, genetic information, and lifestyle factors with unprecedented accuracy. This could lead to earlier disease detection, reduced misdiagnosis rates, and the ability to tailor treatment plans to individual patient profiles.
- Development of new treatment options: AI can uncover hidden patterns and connections that could inform the development of new drugs, therapies, and preventative measures. MDM ensures the data used is accurate and comprehensive, leading to more reliable findings for researchers.
- Improved population health management: MDM systems provide a holistic view of a community's health. This allows healthcare providers to identify at-risk populations, predict potential outbreaks, and allocate resources more effectively.
Conclusion
Accurate, comprehensive, and timely data enables healthcare providers to make informed decisions, personalize patient care, and enhance operational efficiency. AI/ML-powered Master Data Management provides robust solutions for managing and integrating vast amounts of data from diverse sources. These advanced MDM systems ensure that data is not only cleansed and harmonized but also continuously updated and enriched. As a result, healthcare organizations can leverage high-quality data to drive better clinical outcomes, optimize resource allocation, and most importantly, improve the patient experience and satisfaction.
To achieve data-driven excellence, healthcare organizations must invest in MDM solutions enhanced with AI/ML capabilities. These investments streamline data management processes and unlock the full potential of data analytics, paving the way for innovations in patient care and operational efficiency. Watch our on-demand webinar to explore the role of AI-enabled MDM in healthcare.

Michael Ashwell
VP and GM Data Management, Mastech InfoTrellis
Michael is a seasoned professional with over 35 years of experience in enterprise architecture, solution development, cloud offerings, global sales, and consulting. He spent 30+ years at IBM where he held various roles, including leading the Data and Analytics Lab Services Cloud COE, and developed several key offerings.