Table of Content
TABLE OF CONTENTS
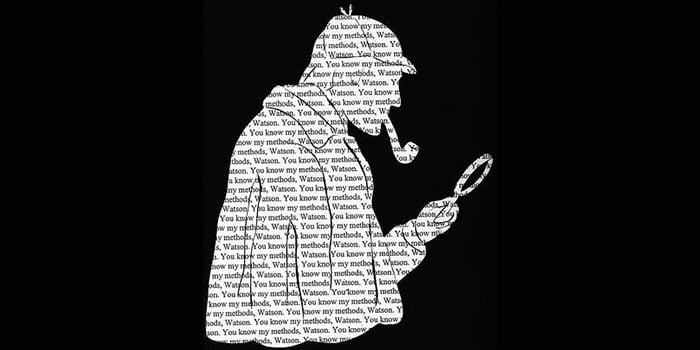
A two-series blog by Prad Upadrashta, Ph.D., Chief Data Scientist, Mastech InfoTrellis
Welcome back! In my last blog, I talked about some of the myths surrounding Data Science and Data Scientists. In this blog, let’s bust some more of them and uncover more brutal truths. Happy reading.
There is undoubtedly a lot of mystique around data science and there is truly a rising demand for competent data scientists. However, just because there is a demand for actual data science, does not imply that merely carrying the label of ‘data scientist’ qualifies one to meet that demand. There is NO demand for unqualified or inexperienced data scientists. However, there is a heavy demand for practitioners who really know what they are talking about. It is a long, hard, dusty trail before one becomes truly a master of the art, at the level of a Sherlock Holmes, …to be considered a data savant, …or a data whisperer. The odds are, one never truly becomes “that good” … in the spirit of the 10,000+ hour rule (courtesy: Malcolm Gladwell). Here, 10,000 hours is perhaps the bare minimum in terms of what it really takes to develop mastery — because a true data scientist sits at the confluence of multiple not-classically-related domains.
Despite all their certificates and coursework, most ‘data scientists’ carrying that moniker seem to have no clue how to make use of statistical concepts to think critically about the data and its meaning in the context of the business domain. I believe the following guidelines comprise a bare minimum of what it really takes to succeed in this business:- You should understand the conceptual universe in which your data and your model live, i.e., the philosophy and beliefs you have about the system you are modeling;
- you should be able to have a reasonably sophisticated discussion about the model with a statistician;
- you should be able to have an informed and strategic/tactical discussion about the methodology and/or code with the technology/infrastructure teams you work with;
- you should be able to have an equally deep discussion about the business with the stakeholders, including a solid understanding of how the model is translated to an action plan that operational people will execute against.
Many data science efforts fail on one or more of these fronts. This is not a failure of data science, but a failure of a cynical community that has failed to invest in the process in the way that it was meant to be leveraged, and therefore, a failure to realize the ROI of real data science. Many organizations think of data science gains in terms of a few percentage points over the status quo – a model that outperforms by 10% or 20% for instance – but I can tell you from personal experience, the ROI from real data science is upwards of 10x – 200x, not merely a few percentage points. That is the real cost of faking your data science. Here, you simply cannot “fake it till you make it.” You can fully expect that if your competition is not faking it, they are making it, and cleaning the floor with what would be your market share.
Re-branding programmers or statisticians or business analysts as ‘data scientists’ will only backfire. You are looking for someone who is fluent in multiple domains and has the expertise and intrapreneurial streak to out compete you, if push came to shove. This kind of instantaneous re-branding is precisely what leads to an inability to follow through on the above. It takes years if not decades of walking the edge along multiple domains before one develops this sort of competency and fluency.
Many do not realize that a model is not merely an equation that describes how an input translates into an output, or some formula for making a prediction with some arbitrary level of accuracy — a model tells you something fundamental about the underlying processes at work giving rise to the observations you see. A model reveals things about the underlying processes at work — both in the way it works, and in the way it fails.
Modeling (hypotheses) should lead to theories, and theories about how things work constitute a science. If you just make statistical predictions without the rest of the context — you haven’t yet done the hard work of translating the business problem into a well-defined scientific framework. You might as well be doing astrology, reading tea leaves, or palmistry — your results will be worth about as much.
The reason organizations are not getting value out of their data, is because they don’t know what value is in that data to begin with, nor do they know what kinds of skills and techniques can be applied to extract the value — save for a few buzzwords. The perceived value of data must be tested against the economic realities of the business model.
Unfortunately, many business leaders are taught to think incrementally forward. But, to do data science correctly and effectively, you need to think non-linearly, often iteratively backwards, and apply that understanding iteratively forwards; while understanding implicitly that future results are not guaranteed to match historical results, and certainly not over the long-term horizon. For instance, model decay is a real thing, and real feedback driven systems will react (sometimes violently) when you try to exploit them or change their equilibrium condition. That additional value has to come from somewhere — usually at the expense of those who are least informed. In poker, if you look around the room and you can’t spot the sucker, it is because you are probably the sucker. So, beware! In that sense, data science is not just important from an offensive viewpoint but is also critical to good defense — or your margins and market share will be eroded by your competition, who are exploiting every trick in the book to take them from you.
If you can reduce your data science to a mundane process of curve-fitting that can be mostly automated using today’s plethora of techniques, you still haven’t got a clue what data science really is — it also means, you really don’t understand the science of your business, in other words, what truly drives your business velocity, volume, customer behaviors, market trends, etc.
In a highly competitive and disruptive world, you will be disrupted eventually if you choose to remain in ignorance of these things — like the taxi business which never predicted that one day that the people who resembled their passengers might become potential competitors! By doing nothing, or sitting on the sidelines, you are not saving money; rather, you are literally betting that nothing will ever change. A historically bad bet.
Read a good Sherlock Holmes novel, and you’ll get a glimpse of how real data science should work in practice. Data Science is not about magically confirming pre-existing biases and acting redundantly — it is about seeing what others are ignoring and exploiting that to drive real additional value. The ability to see around corners is a precious skill.
Discovery takes time, patience, intense focus, deep foundational knowledge, and real abiding curiosity driving multiple lines of attack. To balance this reality against the demands of quarterly reporting and the realities of operating in a matrix environment is no small feat.
There is a heavy demand out there for unicorns like Sherlock Holmes or a Prof. Moriarty. There is not much demand for an inspector Lestrade nor his entire talent pool at Scotland Yard (no offense).
Conclusion
The C-level needs to understand that data science isn’t something done passively with pocket change and a few people with pocket protectors and calculators on someone’s spare time; it is a war-room driven opportunistic exercise of actively defending the business from the competition, creating an impenetrable moat of differentiated value, and waging a war for new revenue. It is a serious enterprise, with serious consequences for the very survival of one’s business.
“The world is full of obvious things which nobody, by any chance, ever observes.” - Sherlock HolmesThe author, “Prad” for short, is a senior analytics executive and experienced data science practitioner with a distinguished track record of driving AI thought leadership, strategy, and innovation at enterprise scale. His focus areas are Artificial Intelligence, Machine/Deep Learning, Blockchain, IIoT/IoT, and Industry 4.0.
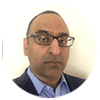
Prad Upadrashta
Senior Vice President & Chief Data Science Officer (AI)
Prad Upadrashta, as Senior Vice President and Chief Data Science Officer (AI), spearheaded thought leadership and innovation, rebranded and elevated our AI offerings. Prad's role involved crafting a forward-looking, data-driven enterprise AI roadmap underpinned by advanced data intelligence solutions.