Table of Content
TABLE OF CONTENTS
- How does Agentic AI work?
- Core principles of Agentic AI
- Key technologies powering Agentic AI
- What are the benefits of Agentic AI?
- Agentic AI vs. Generative AI
- How industries can use Agentic AI
- What business functions can benefit the most from Agentic AI
- Agentic AI vs. AI Agents
- Challenges and considerations
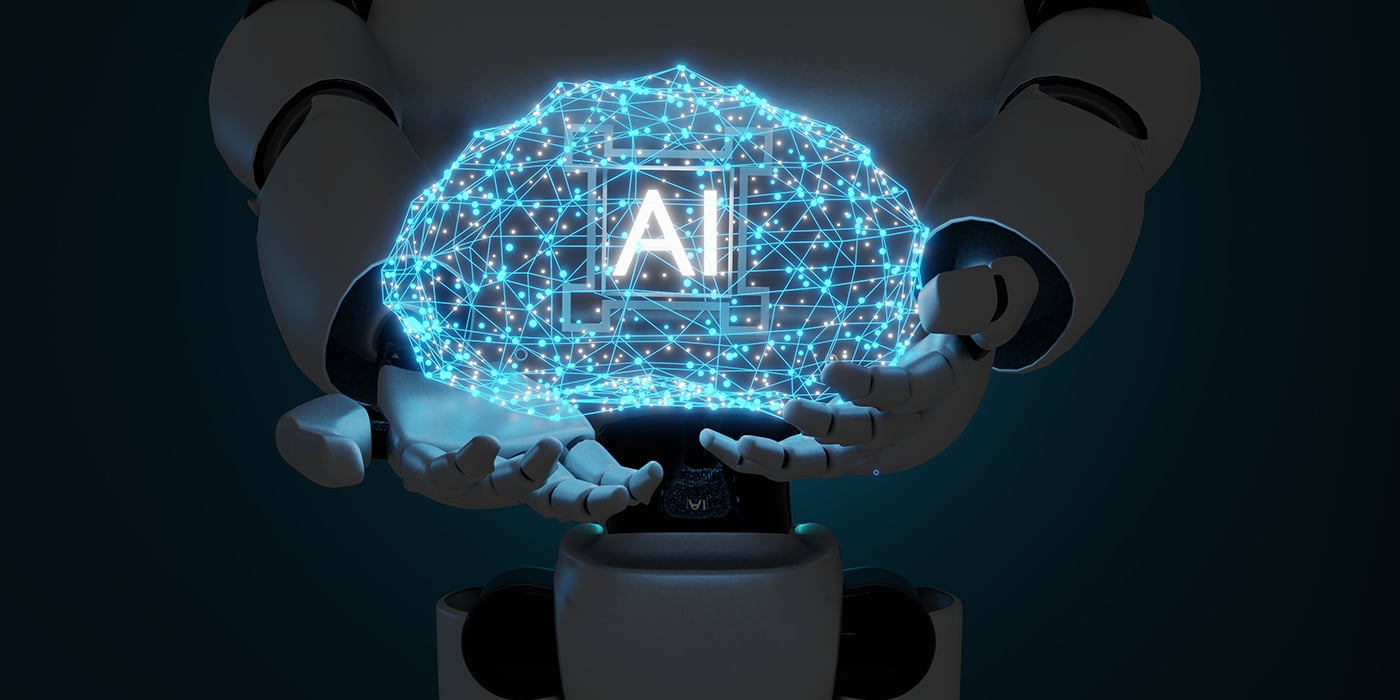
The newest wave of artificial intelligence is here and is set to change the way of decision-making. Agentic AI is the most advanced form of artificial intelligence, characterized by its ability to autonomously plan, execute, and adapt to complex tasks without explicit human direction. In essence, it can do much more than its predecessors.
Agentic AI can make decisions, take actions, and learn independently. It can adapt to new information, solve problems, and collaborate with humans. Agentic AI can be used in many areas, including smart homes, smart manufacturing, customer service, utilities, self-driving cars, and finance.
How does Agentic AI work?
Agentic AI combines traditional AI, like machine learning, with new forms of AI, like large language models (LLMs). Agentic AI is about creating systems that can act intelligently and independently to achieve complex objectives.
- It can use patterns and likelihoods to make decisions
- It can learn and improve through every interaction
- It can work with limited direct human supervision
Core principles of Agentic AI
- Autonomy: This is perhaps the most defining characteristic. Agentic AI is designed to operate with minimal or no human intervention. It can initiate tasks, make decisions, and execute actions independently.
- Goal-orientation: Agentic AI systems are driven by defined objectives. They can understand and pursue specific goals, breaking down complex tasks into smaller, manageable steps.
- Reasoning and planning: Agentic AI can analyze situations, evaluate options, and formulate plans to achieve its goals. This often means using large language models (LLMs) and other AI technologies.
- Context-awareness: It refers to the ability of these systems to sense and comprehend their surroundings, thereby adapting their own behavior while still perceiving and processing information of many forms, namely text, images, and sensor inputs.
- Learning and adaptation: Learning and adaptation allow agentic AI to learn from the experience, gradually making adjustments in its strategies through machine learning techniques and improved performance.
- Interactivity: It allows Agentic AI to communicate, collaborate, and perform actions that involve interacting with humans and other systems.
Key technologies powering Agentic AI
The key technologies powering Agentic AI include machine learning (ML), natural language processing (NLP), large language models (LLMs), deep learning, and real-time data retrieval techniques like Retrieval Augmented Generation (RAG), allowing AI agents to analyze complex data, make autonomous decisions, and adapt to changing situations based on current information.
- Machine learning: The core technology enabling Agentic AI to learn from data, identify patterns, and improve its performance over time without explicit programming for every scenario.
- Deep learning: A subset of machine learning that utilizes neural networks to process complex data, crucial for tasks like image recognition and natural language understanding, which are often used in Agentic AI systems.
- Natural language processing (NLP): Enables AI agents to understand and interpret human language, allowing them to interact with users through text or speech.
- Large language models (LLMs): Powerful AI models capable of generating human-like text, providing insights and responses based on vast amounts of data, which can be incorporated into Agentic AI systems.
- Retrieval augmented generation (RAG): A technique that allows AI agents to access and integrate real-time data from external sources, enhancing their decision-making capabilities by incorporating the most current information.
What are the benefits of Agentic AI?
Agentic AI can revolutionize industries, streamline workflows, and redefine the nature of human-machine interaction. Here are the core benefits of Agentic AI:
- Improve efficiency and scalability
- Free up employees for higher-value projects
- Speed up innovation in scientific discovery
- Lead to more efficient markets and potentially higher returns for investors
In detail:
It can improve efficiency and scalability
Efficiency
- Agentic AI systems are designed to operate autonomously, meaning they can perform tasks without constant human oversight. This reduces the time and resources needed to complete complex processes.
- They can optimize workflows by dynamically adapting to changing conditions and making real-time decisions. For example, an agentic AI could adjust delivery routes based on traffic patterns and weather conditions in supply chain management, minimizing delays and fuel consumption.
- By automating repetitive and mundane tasks, Agentic AI eliminates human error and increases the consistency of outputs. Think of automated data entry or the automatic generation of reports.
Scalability
- Agentic AI can handle vast amounts of data and perform tasks at a scale that would be impossible for humans. This is particularly valuable in industries with high volumes of transactions or data, such as finance or e-commerce.
- Because they are software-based, Agentic AI systems can be easily replicated and deployed across multiple locations or systems. This allows businesses to rapidly expand their operations without hiring and training large numbers of additional employees.
- For example, customer service can be scaled up through agentic AI chatbots that can handle many customers simultaneously, 24/7.
It can free up employees for higher-value projects
Automation of routine tasks
- Agentic AI can take over repetitive, rule-based, and time-consuming tasks like data entry, scheduling, and basic customer support.
- This frees up employees to focus on more strategic and creative work that requires human judgment, problem-solving, and innovation.
Focus on strategic initiatives
- Employees can dedicate their time to developing new products and services, improving customer relationships, and driving business growth.
- They can engage in tasks that require empathy, critical thinking, and complex decision-making, which are areas where humans excel.
Increased job satisfaction
- By eliminating tedious tasks, Agentic AI can make jobs more engaging and fulfilling for employees. This can lead to increased job satisfaction and productivity.
It can speed up innovation in scientific discovery
Data analysis and hypothesis generation
- Agentic AI can analyze vast datasets from scientific experiments, medical records, and research papers to identify patterns and generate new hypotheses.
- This can accelerate the pace of scientific discovery by enabling researchers to explore new avenues of research more quickly.
Automated experimentation
- Agentic AI can design and conduct experiments autonomously, optimizing parameters and analyzing real-time results.
- This can lead to faster discovery of new materials, drugs, and therapies.
Simulation and modeling
- Agentic AI can create complex simulations and models of natural phenomena, such as climate change, disease spread, and astrophysical events.
- This can help scientists to understand these phenomena better and develop solutions to pressing global challenges.
- For example, Agentic AI can simulate the interactions of millions of potential drug candidates with target proteins in drug discovery, significantly shortening the time required to identify promising new treatments.
It can lead to more efficient markets and potentially higher returns for investors
Algorithmic trading
- Agentic AI can analyze real-time market data and execute trades automatically, taking advantage of fleeting opportunities and minimizing human error.
- This can lead to more efficient price discovery and reduce market volatility.
Portfolio management
- Agentic AI can optimize investment portfolios by dynamically adjusting asset allocations based on market conditions, risk tolerance, and investment goals.
- This can lead to higher risk-adjusted returns for investors.
Fraud detection
- Agentic AI can detect and prevent fraudulent activities in financial markets by identifying suspicious patterns and anomalies.
- This can increase market transparency and reduce the risk of financial losses.
Personalized financial advice
- Agentic AI can provide investors with personalized financial advice based on their circumstances and goals.
- This can help investors make more informed decisions and achieve their financial objectives.
- By quickly processing and reacting to market changes, Agentic AI can create a more fluid and responsive investment environment.
Agentic AI vs. Generative AI
Generative AI (GenAI) creates content, while Agentic AI makes decisions and takes actions. Both are types of artificial intelligence (AI) that can improve productivity.
Examples
- GenAI: ChatGPT and Gemini are popular GenAI models that can create text, images, and more.
- Agentic AI: Virtual assistants, copilots, and autonomous vehicles are examples of early-stage Agentic AI.
Can Agentic AI and Generative AI (GenAI) work together?
GenAI and Agentic AI can work together to create solutions that combine creativity and action. For example, GenAI can create marketing copy, and Agentic AI can deploy it to the best channels.
Key points about their collaboration:
- Complementary roles: Generative AI can generate content like text, images, or plans that an Agentic AI system can use to execute tasks or make informed decisions.
- Enhanced capabilities: By combining the creative potential of Generative AI with the decision-making power of Agentic AI, systems can achieve more complex goals and adapt to dynamic situations.
How industries can use Agentic AI
- Automotive: Self-driving cars are a great use case. AI systems learn from driving experiences and adjust behavior to improve safety and efficiency.
- Healthcare: AI agents can help doctors analyze patient data and make better care decisions.
- Supply chain management: AI agents can predict demand, optimize inventory, and coordinate shipments.
- Finance: AI agents can analyze market trends, make trading decisions, and adjust investment strategies.
- Human resources: Agentic AI can automate HR processes like candidate screenings, scheduling interviews, and employee onboarding.
What business functions can benefit the most from Agentic AI
- Customer service: A Generative AI could draft personalized responses to customer inquiries. At the same time, an Agentic AI system could route the queries to the appropriate agent and manage the overall interaction flow.
- Marketing campaigns: Generative AI could generate creative content for marketing campaigns, while Agentic AI could optimize targeting and delivery based on real-time data.
- Business planning: Generative AI could create initial business plans, which an Agentic AI could refine based on market analysis and financial projections.
Agentic AI vs. AI Agents
Agentic AI is a framework that includes AI agents, the individual components that perform tasks within the framework. Agentic AI is the broader concept, while AI agents are the tools that carry out the tasks.
AI agents are software programs that can see what's happening around them, think about it, and then take action to reach a goal. They are powered by technologies like machine learning and language processing to emulate intelligent behavior. Operating with varying degrees of autonomy, from fully independent execution to human-supervised operations, these agents dynamically adapt their responses based on contextual awareness and real-time data acquisition.
Types of AI Agents
- Simple reflex agents
- Model-based reflex agents
- Goal-based agents
- Utility-based agents
- Learning agents
- Hierarchical agents
Challenges and considerations
Key challenges with Agentic AI include ensuring data quality and governance, managing technical complexities, addressing privacy and security concerns, lack of transparency in decision-making, the potential for bias amplification, and the need for effective change management strategies to facilitate employee adoption within an organization when implementing such systems.
Challenges
- Transparency and explainability: Transparency and explainability refer to the fact that, because of complex algorithms and processes employed by an Agentic AI, decisions would be almost impossible to understand, leading to doubts over accountability and much needed trust in the systems.
- Bias amplification: Bias amplification involves situations whereby if training data shows biases an Agentic AI would subsequently exhibit or amplify those biases, resulting in unfair or poorly founded outcomes.
- Data quality and governance: To optimize this kind of system, data quality must be prioritized; this requires effective and sound governing practices that will oversee data collection, cleaning, and subsequent updating.
- Security and privacy concerns: Since Agentic-AI is usually intended to collect and analyze large amounts of data, ensuring the privacy of users and the security of the personally identifiable information that should not be exposed is one considerable challenge.
- Organizational challenges: Common organizational challenges embedded with the implementation of Agentic AI enter the scene with resistance to change, unavailability of efficient skills, and improper resource allocation.
- Monitoring and auditability: Continuous monitoring and auditing are required of each Agentic AI system so that anomalies can be detected, regulated, and corrected whenever needed.
- Technical complexity: Agentic AI systems are very complex since they are advanced, which requires certain levels of technical knowledge renouncing their use in some organizations because of the cost of training.
- Change management: Agentic AI needs to be implemented into the workflows with good planning and change management, and staff has to understand and adapt to the new change.
Key considerations
- Ethical guidelines: The establishment of clear ethical guidelines to circumvent the potential for bias and other unintended consequences from Agentic AI decision-making.
- Human-in-the-loop design: Incorporating human oversight and control mechanisms to review and intervene when necessary.
- Transparency through Explainable AI (XAI): Development of methods making Agentic AI decision-making processes transparent and understandable.
- Data privacy measures: Implementation of explicit and robust data privacy practices to ensure the safeguarding of user information.
- Continuous monitoring and evaluation: Regular monitoring is performed onto the performance of Agentic AI systems to catch unknown issues and provide corrective action.
- Training and education: Providing adequate training for users and developers to understand the capabilities and limitations of Agentic AI.