Table of Content
TABLE OF CONTENTS
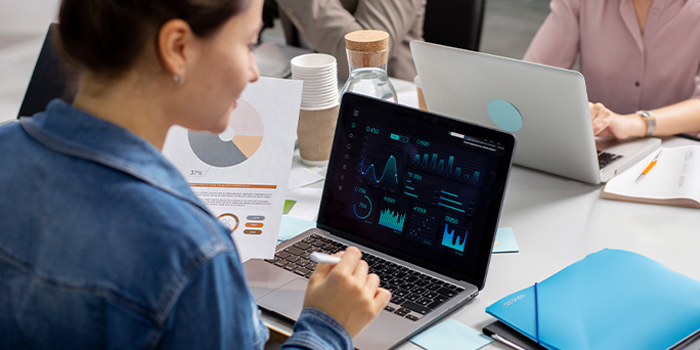
Overview
A recent study by Gartner states that by 2025, graph technology will dominate 80% of data and analytics innovations across diverse industries. Graph technology has revolutionized the field of Artificial Intelligence by addressing some critical problems in knowledge management, supply chain management, financial crime, and more. It offers smarter, better, and more accurate solutions with enhanced performance and greater explainability. Why is graph technology the talk of the data science community? How is it transforming the world of artificial intelligence? So, without delay, let’s delve deeper.
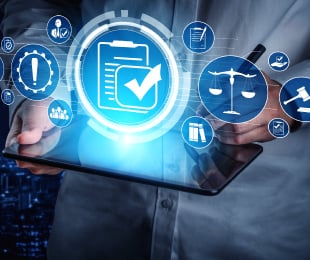
Graph technology: Charting the path to the future
Many enterprises believe that amassing vast volumes of data analytics will inherently result in superior outcomes. By augmenting computational power, they strive to expedite information processing, presuming it will yield profound insights and confer them a competitive advantage for market dominance. However, in most instances, regardless of the degree of value and structure inherent in the data analytics process, a profound understanding of context is indispensable. Comprehending the underlying connections among data patterns makes the pursuit of meaningful insights from the data attainable. This is where graph technologies come into play, helping map data sets and facilitating relationships among them. This strategy allows businesses to identify common patterns, foster contextualization, and create more valuable products to address industrial challenges.
Graph technology: The driving force behind the AI revolution
Graph databases' value and ability to represent and analyze complex relationships between data points are immense. By embracing the concept of graphs in AI, we unlock the enormous potential for leveraging and comprehending the interconnections between entities. This capability becomes crucial in making accurate predictions and informed decisions, as it enables us to harness the power of relationships and uncover valuable insights that drive intelligent outcomes. Here are some techniques in which graph technology contributes to the AI revolution:
- Relationship modeling: Graph technology excels at building relationships between entities. In AI applications, graph databases can capture relations between several data points like concepts, locations, products, or people. As a result, AI systems identify and analyze intricate relationships and use data judiciously.
- Knowledge graphs: Knowledge graphs are a vast repository of structured information across multiple sectors. Knowledge graphs link the information's internal and external data sources, context, and relationships. Knowledge graphs pave the way for AI systems to enhance natural language processing, recommendation engines, and question-answering systems, allowing them to offer more precise and contextually relevant responses.
- Network analysis: Graph algorithms help AI systems analyze the connection between entities by detecting communities, identifying influential nodes, or understanding the flow of information within a network. This graph-based AI capability is applied in fraud detection, cybersecurity, supply chain optimization, social network analysis, and more.
- Graph-based Machine Learning increasing performance: In recent years, Graph Neural Networks (GNNs) have gained immense popularity in machine learning for graph-structured data. GNNs learn from a graph about intricate relationships to classify nodes and make predictions. It offers advancements in social media analysis and drug discovery and drives meaningful insights from unstructured data.
- Greater explainability: Graphs provide visualization and representation of metadata as it flows from data sources to consumption; this aids in AI model decisions. Through visualization, GNNs provide transparent insights into why specific predictions are required. This aspect is valuable in intuitive industries like finance and healthcare, where explainability is significant.
Why is graph AI becoming the "new normal"?
Data in graph technology forms the backbone of the “new normal” existence. Graph-shaped business issues include any case in which one is more concerned with connections among entities than with the entities alone. Graph modeling is most appropriate for complex relationships that are distributed, federated, and flattened compared to hierarchical patterns. Typical graph-based AI applications include:
- Enterprise content management data - Semantic graphs and interlinked metadata can record and manage knowledge across segregated virtual teams.
- Customer transaction data - Graphs can represent relations between consumers and products to suggest offerings of interest in the future.
- The Internet of Things - Graphs can arrange the "objects" like sensor-equipped endpoints for users, industrial, and other applications in non-hierarchical networks of magnificent complexity.
- Linkage analysis - Graphs can irradiate relationships among people, groups, and other shared resources and content. It excels notably in applications such as analysing social networks, fraud detection, and supply chain, where traversals and relationships play pivotal roles.
Conclusion
Graph technology offers a solid foundation for visualizing, analyzing, and leveraging relationships between data entities and efficient data monetization, making it a significant element in changing the AI landscape. Businesses can seamlessly enhance decision-making, identify new opportunities, and develop innovative AI applications by integrating graph-based technologies with other AI tools. Mastech InfoTrellis expertly harnesses graph-based approaches, enabling businesses to drive data-centric decision-making and lead the forefront of AI advancement. As a result, organizations can seize opportunities and foster innovation across diverse industries by unlocking the untapped potential within their data.
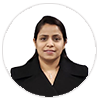
Deepti Soni
Director, Data Science and Analytics
Deepti Soni is a Data Science professional with more than ten years of experience in architecting innovative analytical solutions for strategic business problems.