Table of Content
TABLE OF CONTENTS
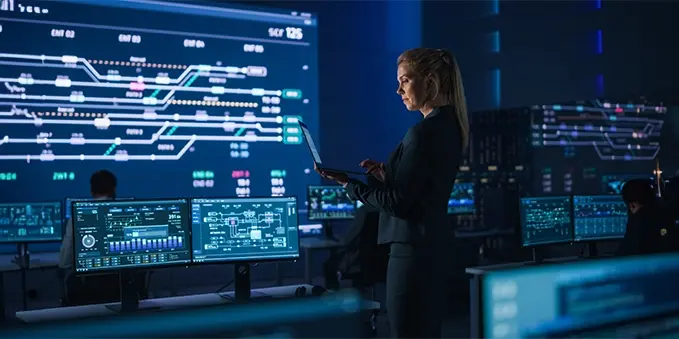
The explosive growth of data has propelled artificial intelligence (AI) to the forefront of innovation. As organizations embrace this digital shift, they increasingly recognize the need for comprehensive governance frameworks to manage and capitalize on the complex interplay of technology and data.
The interplay between data governance and AI presents a compelling narrative of immense potential and profound challenges. Data governance, often perceived as bureaucratic and restrictive, is designed to facilitate the efficient and ethical utilization of data assets. Conversely, AI is a powerful tool that can accelerate the value derived from these data assets. The convergence of these two domains offers a rich landscape of opportunities, necessitating a nuanced approach to managing the inherent risks and maximizing benefits.
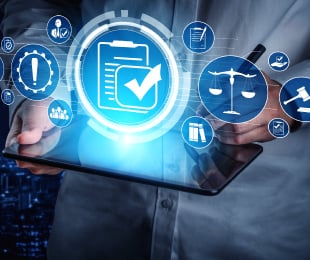
The symbiotic relationship between AI and data governance
Data governance is to data what brakes are to cars. As brakes enable cars to go faster by ensuring control and safety, data governance empowers organizations to harness their data effectively while maintaining compliance and ethical standards. With its ability to quickly process vast amounts of data and derive actionable insights, AI benefits immensely from robust data governance frameworks. Conversely, AI's sophisticated capabilities can enhance data governance practices, making them more efficient and dynamic.
AI's capacity to analyze and interpret large datasets at unprecedented speeds makes it a valuable ally in data governance. For instance, AI-driven tools can automate the classification and tagging of data, ensuring that data is appropriately categorized and accessible. This enhances the quality and consistency of data and ensures that it complies with regulatory standards. By leveraging AI, organizations can streamline their data governance processes, reducing the manual effort required and minimizing the risk of human error.
Governance and AI process traceability – mitigating risk for responsible innovation
Data governance is critical in ensuring traceability throughout the AI development lifecycle. This traceability refers to knowing the entire history of data that trains and operates AI models.
Here's how this helps reduce risk:
- Lineage tracking: Effective data governance practices enable thorough lineage tracking. This means carefully documenting the whole journey of data that trains and operates AI models. This transparency allows organizations to detect any possible biases or errors at each stage of the AI development lifecycle. If an AI output has a problem, traceability allows a focused investigation to find the source of the issue, enabling quick remedial action.
- Model explainability: Data governance frameworks can work with Explainable AI (XAI) tools. These tools provide insights into the reasoning behind AI model decisions. Organizations can assess their validity and mitigate potential risks associated with unexpected outcomes by understanding the factors influencing AI outputs. For instance, if an AI hiring tool consistently favors specific demographics, tracing the decision-making process might reveal biases within the training data. Data governance facilitates the correction of this bias, ensuring fairer AI practices.
In essence, data governance with a focus on traceability empowers organizations to develop and deploy AI responsibly, mitigating risks and fostering a culture of innovation built on trust and transparency.
Addressing bias – a critical challenge
One of the most significant challenges in AI is bias. Bias in AI models often stems from biases present in the training data, which can lead to skewed outcomes and perpetuate existing inequalities. Semantic models, which focus on the meaning and context of data, offer a sophisticated approach to addressing this challenge. These models can help identify and mitigate biases in training datasets by providing a deeper understanding of data.
The impact of bias on decision/output accuracy
AI systems rely on the data they are trained on to make predictions and decisions. If this data is biased, the system's predictions and decisions will also be biased, leading to inaccurate outcomes. For example, facial recognition technology has been shown to have higher error rates for individuals with darker skin tones due to biased training data. This inaccuracy can result in wrongful identifications and significant legal and ethical implications.
Furthermore, biased AI models can propagate and exacerbate existing societal inequalities. For instance, an AI system used in lending might deny loans to individuals from certain minority groups based on biased historical data, thereby perpetuating economic disparities. Accurate and fair AI decision-making is crucial for fostering trust and ensuring that AI systems benefit all users equitably.
The regulatory perspective on bias
Bias in AI systems is a significant concern from a regulatory perspective. Regulatory bodies worldwide are increasingly scrutinizing AI systems for fairness and transparency. For instance, the European Union's General Data Protection Regulation (GDPR) includes provisions for data fairness. Organizations must ensure automated decision-making processes are free from bias and discrimination.
Failure to address bias in AI systems can result in severe legal and financial consequences. Organizations using biased AI systems may face hefty fines, legal battles, and reputational damage. For example, the New York City Council passed a law requiring companies to audit their AI hiring tools for bias, reflecting a growing trend toward regulatory oversight of AI technologies.
Bias in AI models is a multifaceted challenge that impacts result efficiency, decision accuracy, and regulatory compliance. Addressing this issue requires a sophisticated approach, leveraging semantic models and metadata to understand data better and mitigate inherent biases. By doing so, organizations can enhance the fairness and accuracy of their AI systems, ensure regulatory compliance, and ultimately foster more equitable and effective decision-making processes. Strong data governance practices help identify and mitigate biases in datasets and enable using diverse and representative datasets, ensuring fair and unbiased AI models.
Additionally, semantic metadata is crucial in uncovering and rectifying inherent biases. By incorporating semantic metadata, organizations can enhance the fairness and accuracy of AI models, supporting more equitable decision-making within the data governance framework.
Balancing privacy and insight
In an era where AI systems are revolutionizing various industries, the potential for these powerful tools to access and process vast amounts of data, including highly sensitive personal information, raises significant concerns. Data privacy in AI models is no longer just a compliance issue but a critical factor for building trust, mitigating risks, and ensuring ethical development.
Here's why data privacy in AI models is paramount:
- Building trust and transparency: Consumers are increasingly wary of how AI systems collect, use, and potentially share their data. Upholding data privacy fosters trust and transparency with users, ensuring they feel comfortable interacting with AI-powered applications. This is especially important in sensitive sectors like healthcare or finance, where data breaches or misuse of information could have severe consequences.
- Mitigating reputational risk: Breaches of personal data or misuse of information for AI development can lead to significant reputational damage for organizations. Strong data privacy practices minimize these risks, protecting brand reputation and fostering long-term customer trust.
With a growing number of regulations worldwide addressing data privacy in AI, organizations must prioritize this aspect to comply with legal requirements like GDPR and operate ethically and responsibly within the AI ecosystem.
Data governance policies enforce strict data security and privacy measures, including protecting sensitive data used in AI models, ensuring compliance with data protection regulations (e.g., GDPR, CCPA), and implementing robust access controls and encryption. Semantic models also contribute to data anonymization processes, which are crucial for preserving privacy. Organizations can implement measures that anonymize data without compromising its utility by understanding the semantic context of data. This ensures that insights can be drawn from the data without revealing personal information, reinforcing data governance's confidentiality aspect.
A Gartner report indicates that 75% of the world's population will have their data protected under privacy laws by 2024. As privacy regulations are expected to expand significantly across multiple jurisdictions in the next two years, organizations are prioritizing their privacy program efforts. By leveraging semantic metadata, these organizations can navigate complex regulatory environments more effectively, ensuring that their AI systems comply with stringent privacy standards while delivering valuable insights.
The dual role of AI in data governance
AI serves both as an enabler and a consumer of data governance. Organizations with solid data governance foundations are better positioned to exploit AI technologies. Data governance ensures that AI models comply with industry-specific regulations and enforce strict data security and privacy measures, protecting sensitive data used in AI models and reducing legal risks. Strong data governance policies enable the ethical use of AI with well-defined policies and documentation. These organizations can leverage AI to enhance their data governance practices, leading to more accurate and reliable data management.
Conversely, AI can catalyze organizations with weaker data governance frameworks, pushing them to adopt more comprehensive and sustained data governance practices. AI can automate compliance checks against regulatory standards, ensuring continuous adherence and flagging potential issues. For example, AI-powered data governance tools can automate data classification, metadata management, data cleansing, and validation tasks. This improves the efficiency and accuracy of data governance processes and frees up resources for more strategic initiatives. As a result, organizations can achieve a more holistic approach to data governance, ensuring that their data assets are managed effectively and ethically.
The 2024 McKinsey Global Survey on AI highlights a significant surge in generative AI adoption, with 65% of respondents reporting regular use within their organizations. These figures underscore AI's transformative potential in enhancing data governance and driving business value.
Conclusion
The intersection of data governance and artificial intelligence represents a paradigm shift in how organizations manage and utilize their data assets. By leveraging AI, organizations can enhance their data governance practices, ensuring that data is managed efficiently, ethically, and in compliance with regulatory standards. At the same time, robust data governance frameworks are essential for maximizing the value of AI technologies, ensuring that they are used responsibly and effectively.
Addressing challenges such as bias and privacy requires a sophisticated approach, with semantic models and metadata playing a pivotal role. As organizations continue to navigate this complex landscape, the symbiotic relationship between AI and data governance will be crucial in driving innovation, enhancing operational efficiency, and ensuring ethical data practices.
The future of data governance lies in embracing AI technologies, not as a replacement but as a powerful ally in the quest for better data management and utilization. If you want to explore more about robust data governance in the AI era, understand its impact on regulatory compliance, internal efficiency, and maximizing the value of your data assets, watch our on-demand webinar where our data experts will share a practical framework for building a successful data governance program, leveraging automation and AI effortlessly.
Tags
Data Governance

Michael Ashwell
VP and GM Data Management, Mastech InfoTrellis
Michael is a seasoned professional with over 35 years of experience in enterprise architecture, solution development, cloud offerings, global sales, and consulting. He spent 30+ years at IBM where he held various roles, including leading the Data and Analytics Lab Services Cloud COE, and developed several key offerings.