Table of Content
TABLE OF CONTENTS
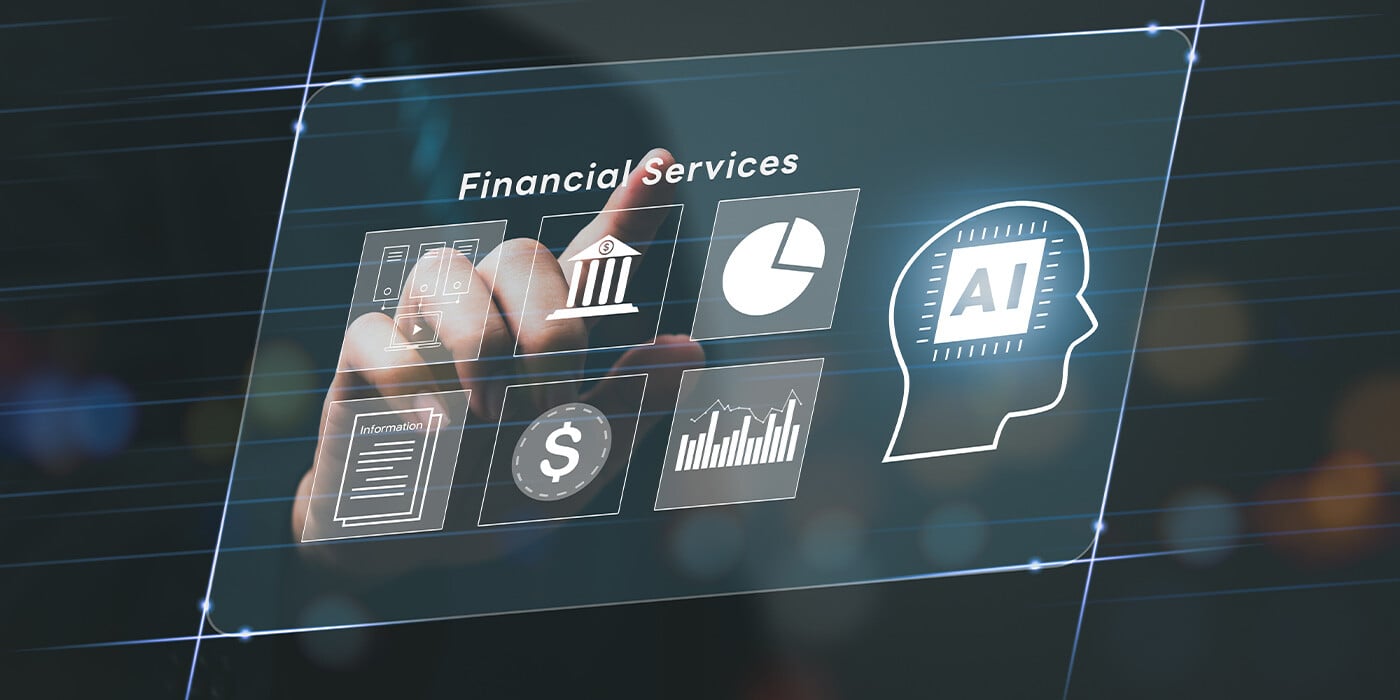
The financial services industry BFSI (Banking, Financial Services, and Insurance) is undergoing a significant transformation driven by the power of artificial intelligence (AI). At the forefront of this transformation is the application of AI-driven analytics, which leverages intelligent analytics to deliver highly personalized financial services. By integrating smart data analytics and machine learning (ML) analytics, BFSI organizations can enhance customer experiences, optimize operations, and drive profitability.
This article explores the transformative potential of AI-driven analytics in personalizing financial services and examines the core benefits, key techniques, and implementation considerations for BFSI institutions.
Why personalization matters
Customizing products and services has become essential to meet the needs of tech-savvy customers who desire personalized solutions. Old-school methods of segmentation and targeting haven't kept up with what today's customers want. This has opened the door for AI-powered analytics to tackle this growing issue.
AI-driven analytics leverages intelligent tools like machine learning, augmented data analytics, and automated data analysis to deliver hyper-personalized services that redefine customer experiences.
- Shifting customer expectations: The digital revolution has changed what customers want. People now expect smooth and easy-to-use services just like what big tech companies such as Amazon and Netflix offer. In the banking and finance world, this means giving custom-made advice, suggesting products that fit each individual, and proactive support.
- Challenges of generic financial solutions: Generic financial products often fail to address the nuanced needs of individual customers, leading to dissatisfaction and churn. Personalization enables these institutions to offer solutions that resonate with each customer's unique financial journey.
- Competitive edge through hyper-personalization: The personal touch also gives firms a competitive edge as they are able to foster deeper customer relationships, increase brand loyalty, and improve lifetime value. In the heavily saturated BFSI industry, AI is helping firms stand out in an already competitive market.
Key components of AI-driven analytics in financial services
Intelligent analytics
Intelligent analytics joins AI with traditional business intelligence built on actionable insights. This does not limit itself to descriptive power but does include predictive and prescriptive capabilities that result in banks being allowed better decision-making capabilities.
Example: Banks use intelligent analytics to predict customer churn and implement retention strategies proactively.
Machine learning analytics
ML analytics identifies patterns and trends in data. This enables BFSI organizations to accurately forecast customer behavior, market movements, and risk factors.
Example: Credit scoring models use machine learning to assess the creditworthiness of loan applicants.
Augmented data analytics
Augmented data analytics integrates AI, machine learning, and natural language processing (NLP) to automate data preparation, analysis, and visualization. This accelerates the time to insight and reduces dependency on data scientists.
Example: Insurance companies use augmented analytics to evaluate claims data and detect fraudulent activities.
Automated data analysis
Automated data analysis optimizes the process of extracting insights from raw data, enabling real-time decision-making. This allows organizations to focus on strategic initiatives by eradicating manual data processing.
Example: Investment firms use automated analytics to monitor portfolio performance and suggest rebalancing strategies.
Advanced analytics with AI
Advanced analytics with AI encompasses techniques such as deep learning, predictive modeling, and sentiment analysis. These methods provide a deeper understanding of customer needs and market dynamics.
Example: Banks use sentiment analysis to gauge customer satisfaction through social media interactions and feedback.
Core benefits of AI-driven analytics in financial services
AI automates complex data analysis tasks and uncovers valuable insights from unstructured data. Augmented data analytics allows organizations to mix conventional data processing methods with AI analytics to achieve more significant efficiency and precision.
Key benefits include:
Enhanced customer experience (CX)
- Personalized recommendations: AI can process customer data, and suggest, for example, loans, investments, or an insurance policy, which are in line with the customer's profile and risk profile.
- Proactive customer service: AI chatbots can offer continuous customer support, quickly answer questions, and effectively resolve problems.
- Improved customer segmentations: AI can segment customers into groups based on demographic, financial, and risk profiles, allowing the execution of personalized marketing and product offers.
Increased operational efficiency
- Fraud detection & prevention: AI algorithms can work on-the-fly transacting data in order to detect & prevent fraud, protect customer money, and minimize financial loss.
- Risk management: AI can assess creditworthiness, market risks, and operational risks more effectively, allowing for informed decision-making and proactive risk mitigation strategies.
- Automation of processes: Repetitive tasks like data entry, report generation, and customer onboarding can be automated using AI, freeing up human resources for more strategic activities.
Key AI-driven analytics techniques in BFSI
Several AI techniques are transforming financial services through advanced data analytics. Here are a few prominent examples:
Predictive modeling
- Predicting customer churn: AI models would help institutions determine which customers are most likely to leave, so they can implement retention strategies on them.
- Credit scoring and risk assessment: AI may develop more precise models for credit risk than the ones currently used, promoting better lending decisions.
- Market trends forecasting: The ability of AI to analyze market data for predicting trends would enable investment strategies to be adjusted accordingly.
Natural language processing (NLP)
- Sentiment analysis: By analyzing customer reviews and social media discussions through natural language processing, sentiment is gauged and areas of improvement identified.
- Chatbots & virtual assistants: NLP empowers chatbots to understand the natural language used by customers, respond satisfactorily to their queries, and provide the personalization that customers expect.
Computer Vision
- Fraud detection: AI can analyze images and videos to detect fraudulent activities in transactions or documents related to loan applications or insurance claims.
- KYC/AML compliance: AI can automate Know Your Customer (KYC) and Anti-Money Laundering (AML) processes by analyzing documents and identifying suspicious patterns.
Use cases
- Personalized wealth management: AI analytics allows financial advisors to offer customized investment portfolios based on individual client profiles, goals, and risk tolerance.
- Real-time fraud detection: AI algorithms analyze transaction data to detect and prevent fraudulent activities in real-time, reducing financial losses and enhancing customer trust.
- Credit risk assessment: ML models evaluate credit risks more accurately by analyzing a combination of traditional financial data and alternative data sources, such as social media behavior.
- Dynamic pricing in insurance: Advanced analytics enables insurance companies to offer dynamic pricing models based on customer behavior, driving competitive advantage.
- Enhanced customer support: AI-powered chatbots and virtual assistants use natural language processing to provide personalized assistance, improving customer satisfaction and reducing service costs.
Implementing AI-driven analytics
Various factors should be kept in mind while successfully deploying AI-driven analytics in the BFSI:
Data preparation & management
- Data quality: It is very important that there is data accuracy, completeness, and consistency in order to develop reliable AI models.
- Data security and privacy: Protecting customers' private information necessitates very high data security measures and protection.
- Data governance: There should be clear policies and procedures to regulate the proper usage of data.
Building & deploying AI models
- Model development: Careful selection of inference models according to the correlative data set is primordial in procedures.
- Model validation and testing: A severe check involved, suitably bringing out the efficiency in accuracy, reliability, and generalizability upfront.
- Model deployment and monitoring: Getting models into production and then continuously monitoring to allow optimization and customer-centeredness.
Challenges and considerations
Although AI-based analytics is a vast opportunity, there are challenges that must be addressed.
- Data quality issues: Data quality issues, such as missing values, data inconsistency, and biases, can impede model performance.
- Model explainability: This is the most important aspect in creating the trust of customers and regulating agents in the models used in AI.
- Ethical considerations: Priorities include ethical issue resolution around data privacy, AI biases, and fairness.
- Regulatory compliance: Compliance with relevant regulations governing data usage in the financial sector and implementation of AI models is causing headaches.
The future of AI-driven analytics in BFSI
New tech like edge computing, blockchain, and quantum computing could make AI even better and open up fresh possibilities. Edge computing lets AI work in real time at the network's edge cutting delays and boosting performance. Blockchain can boost data safety and openness, while quantum computing can speed up the creation and use of more complex AI models.
The growth of AI-driven platforms and ecosystems is also changing the BFSI scene. These platforms allow AI solutions to work across the whole value chain, from signing up customers to managing risks and investments. This helps new ideas and teamwork flourish in the ecosystem speeding up the use of AI-based solutions.
Coming up with new ideas all the time is key to staying on top in this fast-changing scene. Banks and financial companies need to put money into research and new product development. They should create an environment that encourages fresh thinking. They also need to team up with tech firms to make the most of the newest breakthroughs in AI.
Conclusion
AI-powered analytics has a big impact on the banking world. It's making things more personal, faster, and safer for customers. Banks and other financial institutions can get a lot of value by using AI tools like smart data analysis, machine learning, and NLPs.
But to make it work well, they need to deal with some tricky stuff. They have to make sure their data is good, explain how their AI makes decisions, and follow all the rules. If these businesses use AI in a smart and honest way, they can build trust, be open about what they're doing, and stick to the rules. At the same time, they can come up with new ideas and grow bigger.
As the banking and finance world keeps changing, teaming up with experts like Mastech InfoTrellis can help companies make the most of AI-powered analytics. Mastech InfoTrellis offers custom solutions and the latest tech to support banks and financial firms. This enables them to provide great customer service, improve how they work, and stay on top in a tough market.